
As organizations globally rush to harness the power of artificial intelligence (AI), many are overlooking a crucial step: the accurate curation and preparation of their data. This oversight is emerging as a significant trend among large organizations, according to Dave Curtis, Chief Technology Officer at RobobAI, a global fintech company specializing in AI-driven ethical supply chain transformation.
Curtis points out that a primary reason for the failure of many AI projects is the unexpected costs associated with data collection and rectification. ‘Accurate and complete data is the foundation of all analytics on which business decisions are made,’ he states. The importance of this foundation becomes even more critical when considering that AI predictive models rely heavily on extensive historical data to forecast future trends.
Many companies are grappling with poor data quality, Curtis notes. This issue stems from various factors, including multiple sources of truth, lack of automation or validated sources, and errors from manual data entry. These data quality problems create substantial barriers to effective decision-making based on the data.
To address these challenges, RobobAI is observing an increase in the use of AI for data improvement rather than predictive modeling. Curtis explains that organizations are exploring ways to leverage AI to reduce the manual effort required in data preparation and correction. ‘Companies are exploring where they can get demonstrable ROI by reducing or removing this effort,’ he says.
RobobAI’s platforms exemplify this approach, utilizing AI techniques such as Natural Language Processing (NLP) and clustering to preprocess data. These methods help identify and reduce duplication while enhancing data records by filling in missing attributes from other sources.
Curtis emphasizes the importance of maintaining data quality once it has been corrected. Many organizations currently dedicate entire teams to data fixes, a resource-intensive approach that AI could potentially streamline or replace.
The focus on analytics and AI is widespread, but Curtis argues that organizations need to prioritize their data foundations first. ‘Companies need to consider the entire end-to-end model when building a case and understanding the potential returns,’ he advises.
This trend highlights a critical juncture in the AI adoption journey for many organizations. As they seek to leverage AI for competitive advantage, the quality and preparation of their data emerge as key determinants of success. The insights from RobobAI suggest that AI itself may play a crucial role in preparing data for more advanced AI applications, creating a virtuous cycle of improvement and innovation.
For organizations looking to implement AI solutions, the message is clear: invest in data quality and preparation as a foundational step. By doing so, companies can avoid unforeseen costs, improve the accuracy of their AI models, and ultimately derive greater value from their AI initiatives.
As the AI landscape continues to evolve, the ability to effectively prepare and manage data will likely become an increasingly important competitive differentiator. Companies that master this aspect of AI implementation may find themselves better positioned to reap the benefits of AI-driven insights and automation in their operations and decision-making processes.
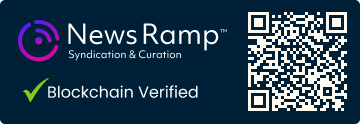
This news story relied on a press release distributed by 24-7 Press Release. Blockchain Registration, Verification & Enhancement provided by NewsRamp™. The source URL for this press release is RobobAI CTO Emphasizes Importance of Data Preparation for AI Success.